Sharing an informative article by Sarah McKenna (CEO of Sequentum & Forbes Technology Council Member), The Importance Of Transparency And Trust In Data And Generative AI. It includes factors for responsible data collection (aka scraping) and web data usefulness for AI post processing. She touches on security, adherence to regulatory requirements, bias prevention, governance, auditability, vendor evaluation and more.

In the age of data-driven decision-making, the quality of your outcomes depends on the quality of the underlying data. Companies of all sizes seek to harness the power of data, tailored to their specific needs, to understand the market, pricing, opportunities, etc. In this data-rich environment, using generic or unreliable data not only has the intangible costs that prevent companies from achieving their full potential, it has real tangible costs as well.
Bad Data is Costly
While this is an obvious statement, the amount and the extent of the cost of bad data is not. According to a survey by research firm Gartner, “organizations believe poor data quality to be responsible for an average of $15 million per year in losses.” In the same study, Gartner also found that “nearly 60% of those surveyed didn’t know how much bad data costs their businesses because they don’t measure it in the first place.” This underscores the critical need for businesses to ensure the accuracy and reliability of their data to drive informed decision-making and mitigate potential liabilities.
Sources & Risks of Data
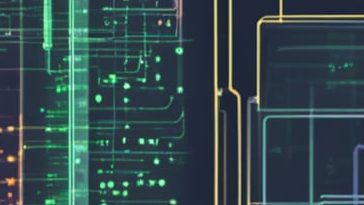
Companies draw data from a myriad of sources including internal operations, customer insights, market research, financial records and data scraped from the web. However, utilizing web-scraped data presents specific challenges:
• Accuracy And Reliability: Web-scraped data quality can vary significantly across different platforms. Some data providers offer a “black box solution” with little to no insight as to how the data is sourced or what happens to the data between sourcing and delivery.
• Privacy And Security: Collecting web-scraped data requires knowing and following all data privacy and compliance regulations. Privacy laws dictate how personally identifiable information is gathered, stored, used and shared. These laws also vary by state and by country; the EU is especially restrictive on the use and even storage of data.
• Adherence To Regulatory And Corporate Requirements: Companies need to follow government laws and their own corporate guidelines to avoid fines and legal proceedings, as violators may face additional legal liability in private lawsuits. For example, failure to have a systematic process to identify and track compliance risks to adhere to Section 204A of the SEC Code of Ethics Rule 1.Violations for civil penalties state that individuals could be subject to civil penalties of up to $1 million or 3 times the profits or losses, whichever is greater (under US rules).
• Complexity And Bias: Managing vast and complex web data requires rigorous governance to address potential biases and ensure relevance. Bias can occur from the outset when framing a question to be solved, to how data is collected and organized. Recognizing the potential for bias is the first step to ensuring it’s addressed, so the data yields optimal, unbiased results.
• Integration And Interoperability: Web-scraped data comes from diverse sources and formats, making it challenging to integrate with internal data systems. The ability to ingest data from any source, configure it to meet specific needs and deliver it in the desired format with reliability is the objective. This requires significant attention to detail when crafting the collection strategy as well as a technical facility to work with any web-based interface, protocol and content format.
• Governance And Compliance: Companies must establish clear policies and procedures for collecting, storing and using web-scraped data to ensure legal and regulatory compliance. Failure to adhere to data governance principles can result in legal, reputational and financial consequences.
• Observability And Auditability: It’s not enough for you to have transparency end to end, to be positioned for both today’s legislation and tomorrow’s that will likely include increased AI regulation. Every step and interaction along the entire data journey needs to be auditable.
Vendor Considerations
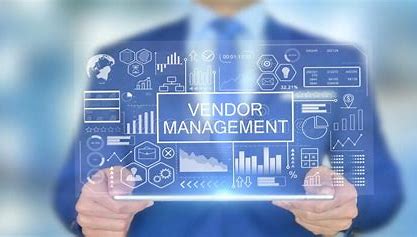
Whether sourcing data internally or from external vendors, businesses must vet suppliers rigorously for the benefit of ensuring trust in the data across all users and, again, to avoid legal or reputational consequences.
• Reputation And Compliance: Assessing vendors based on reputation, experience and adherence to data collection standards ensures reliability and legality of sourced data. This consideration should include legal and compliance.
• Quality Assurance: Vendors should provide detailed quality assurance processes and audit trails to guarantee data integrity. The data team is ultimately responsible but follows legal and any company guidelines.
• Transparency And Documentation: Clear ability to document data sources, collection methods and transformation processes will help you ensure transparency and accountability.
• Auditable Processes: Documented audit trails to allow stakeholders to trace data from its origin to its application, ensuring reliability and compliance with evolving regulations. This is also a consideration for legal and compliance.
• Integration And Validation: The ability to integrate web data with internal systems requires input from current systems in use across the organization. Additionally, validation mechanisms are required to verify data accuracy and relevance. This consideration should include input from information technology and business analysts with an understanding of the user requirements.
• Experience In AI: Companies that proclaim they’re AI experts are abundant. Not just companies who understand current limitations such as AI hallucinations. Companies who have worked with AI to specifically enrich data, ethically, responsibly and with an eye to the future are limited. Most are focused on presenting a result, a black box solution, not on documenting every interaction with the data. The inability to show the value your company added to enrich data with AI will limit future financial compensations for any transformational work completed.
Building a Culture of Trust
Incorporating stakeholders from analysts to executives early on in data governance processes fosters a culture of transparency and accountability. This approach ensures that decision-makers understand data limitations, origins and implications, thereby enhancing trust in decision-making outcomes.
Conclusion
The path to leveraging data and AI lies in transparency, trust and governance. This path involves navigating the inherent complexities in order to make optimal, informed decisions and mitigate risks for competitive advantage.
By prioritizing and embracing these key principles and infusing them into every step, businesses can enhance their opportunities and outcomes while mitigating risk.

(1) When SEC regulators come knocking you have to be able to provide an evidenced based audit proving you not only have a code of ethics specified but that you are following it. If your operation involves black box scraping services or remote engineers writing python code and manipulating data on unmanaged machines, the SEC regulator may decide to issue hefty fines. It’s ok to have mistakes as long as there is a clear process which is memorialized in writing and evidence that it is being followed.